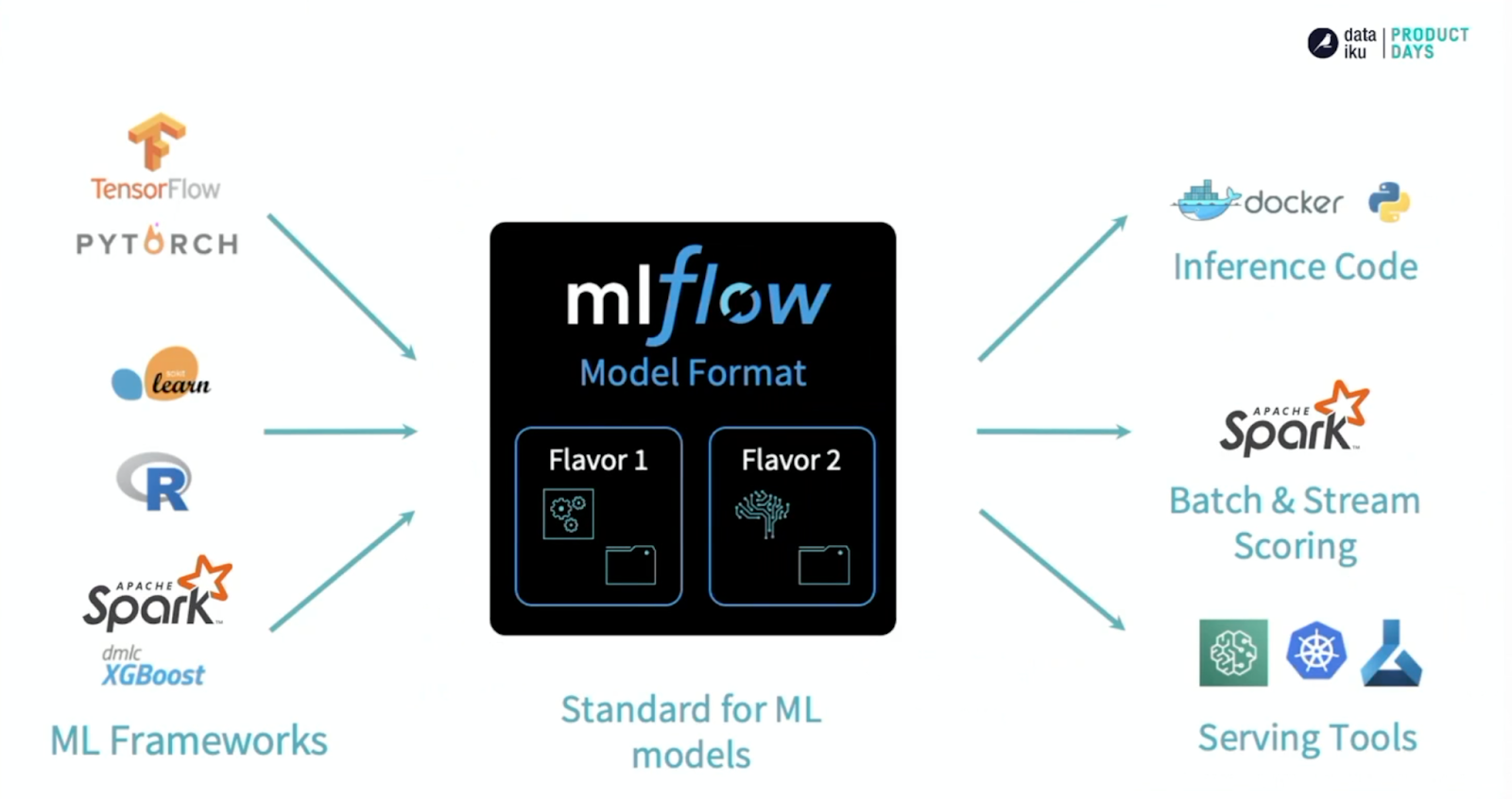
Thought Leadership
Streamlining MLOps with Dataiku, MLFlow, and Sagemaker
Data scientists often grapple with the challenge of transitioning their models between different environments, like moving from Amazon Web Service (AWS) to a tool like Dataiku. Fortunately, Dataiku understands these concerns and has taken significant steps to simplify the process. Dataiku highlighted the ease of this process at Everyday AI’s Tech Day.
MLFlow and Sagemaker Integrations: Bridging the Gap
In a session on MLFlow and Sagemaker integrations, Dataiku emphasized its commitment to addressing this common pain point. The session underscored three key ingredients for a successful MLOps program: Unify, Operationalize, and Repeat.
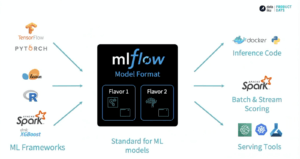
Unify: Dataiku advocates for a single environment for both development and operations. This unification minimizes the need for extensive rework when transitioning models, resulting in faster time-to-value.
Operationalize: Automation is a cornerstone of an efficient MLOps program. Dataiku simplifies the development process, reducing the manual effort required to make models production-ready.
Repeat: MLOps is a continuous process, not a linear one. Dataiku ensures that the model transfer process remains seamless as you iterate on your data projects. With a data science team working in Dataiku, their models can be re-used across multiple projects and applications.
Easily Deploy Your Data Science Model Anywhere
Dataiku’s commitment to easing the model transition process is further exemplified by its deployment flexibility. You can deploy your models from a variety of platforms, including Azure, Vertex AI, SageMaker, Databricks, and Snowflake.
But what sets Dataiku apart are the benefits it offers during the development and deployment stages. Here’s how Dataiku makes it easy:
– Bring Your Own Code: You can seamlessly import you existing code into Dataiku, minimizing the need for extensive rework.
– Model Explainability Charts: Dataiku provides tools for model explainability, helping data scientists understand and interpret model outcomes.
– Single-Click Deployment: You can deploy your models with just a single click, reducing the complexity and time required for production deployment.
Effortless Model Transfer with MLFlow and Sagemaker
Dataiku’s integration with MLFlow and Sagemaker is a game-changer. With MLFlow, you can build models inside or outside of Dataiku and seamlessly import them without the need to update code. MLFlow even allows you to output results directly to a Managed Folder, enhancing collaboration and data accessibility.
Sagemaker integration is equally impressive. Models can be easily deployed and transferred between Dataiku and Sagemaker, and an IAM (Identity and Access Management) role is used to sync between these environments. This means you can leverage any Sagemaker endpoint and update model versions and parameters with ease.
One critical point to note is the importance of making testing data available from Sagemaker to Dataiku. Ensuring this data flow is vital for maintaining model integrity throughout the transfer process.
Effortless Model Comparisons
Finally, Dataiku simplifies the model comparison process. Data scientists can easily compare key metrics like ROC (Receiver Operating Characteristic), AUC (Area Under the ROC Curve), Accuracy, Recall, Lift, and more across different models. Additionally, comparing ROC curves is made straightforward, allowing data scientists to make informed decisions about model performance.
Dataiku has placed a strong emphasis on making it easy to import and export models, reducing the need for extensive rework. With seamless integrations, simplified deployment, and comprehensive model comparison tools, Dataiku empowers data scientists to focus on what they do best: driving insights and value from their data projects.
See Also: