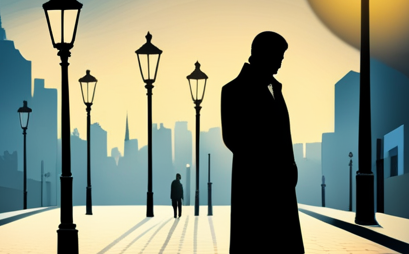
Analytic Insights
Searching Beyond the Lamp Post: Finding the Right Data Talent
In the data world, the term “unicorn” refers to a highly sought-after employee with a rare combination of skills across diverse yet related job types. Much like their mythical counterparts, these professionals are both exceptional and scarce.
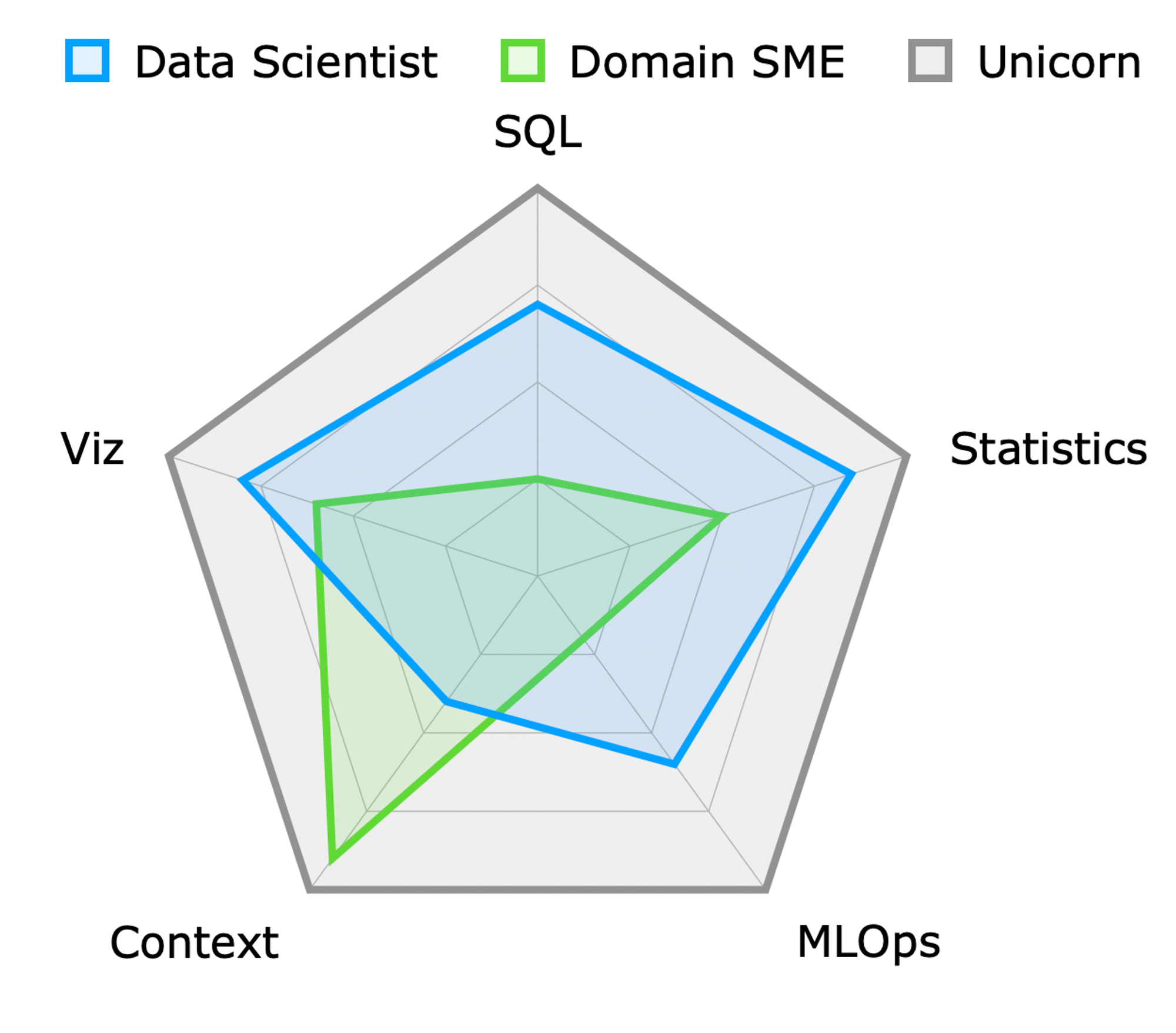
This concept of rarity and versatility leads us directly to the nature of specialists. Specialists are sometimes described as T-shaped, possessing deep skills in one area and broader, more superficial knowledge across other related areas.
This mix of depth and breadth is crucial when it comes to effective project staffing. At the risk of turning human beings into Tetris blocks, one of the challenges I face in selling and scoping projects is figuring out staffing. My preference is always for very small, skilled, and highly experienced teams.
Project Staffing
The negative impact of inexperienced analysts and data engineers on projects, often due to a few inadvertent mistakes, highlights the importance of carefully selecting the right team members. This is why smaller, more experienced teams are preferred — to reduce the risks of communication errors and administrative overload.
The scoping and staffing stage of machine learning projects is particularly critical. It requires careful anticipation of communication cycles and the collaboration time needed across different skill sets.
Project success often hinges on recognizing the inherently iterative nature of data analysis, especially in feature engineering for modeling. Project timelines and budgets can be severely impacted by:
Lack of internal time allocation:
– Hiring consultants is not the same as hiring staff augmentation or temporary employees. You need to plan to dedicate substantial amounts of time partnering with them to bring organizational and domain expertise to their process and tools knowledge.
Long communication cycles and “Game of Telephone” signal distortions:
– Specialists don’t usually build a holistic view of the value proposition and can’t make minor decisions on their own which would be intuitive to an employee or domain expert. If your data scientist isn’t doing the data prep and engineering, then you need to plan for long cycles. Yes, you may be able to run some auto ML for hyperparameter tuning, but there’s no substitute for collaboration and deep domain understanding for feature engineering.
The Drunkard’s Walk
The analogy of the drunkard searching for their lost keys aptly describes the dilemma in data project management. Just as a drunk might search for their keys under a lamp post, because that’s where the light is, project managers might limit their search for insights to the data that is easily accessible, rather than where the most valuable insights might be found. It is similarly futile to think that the existing dimensions in your organization’s data will meet the needs of ML models, given that the traditional data that’s been generated and stored was likely operational, not analytical.
Project Execution and Budgeting
Feature engineering and data preparation are critical in project execution, demanding both technical skill and domain expertise. The iterative nature of these processes emphasizes the need for adaptable and knowledgeable team members.
Underestimating the resources and time required for a project is a common issue. A realistic approach to budgeting and timeline planning must consider the exploratory and iterative nature of data analysis.
It is essential to seek out or create the data that will truly inform business decisions. This approach should be integrated into every aspect of project planning, from team composition to budgeting to timeline estimation.
If you want to use only the data you have in its current structure and formats, you’re searching for your keys not where you likely lost them, but under the lamp post, and only a fool or a drunkard would do that.