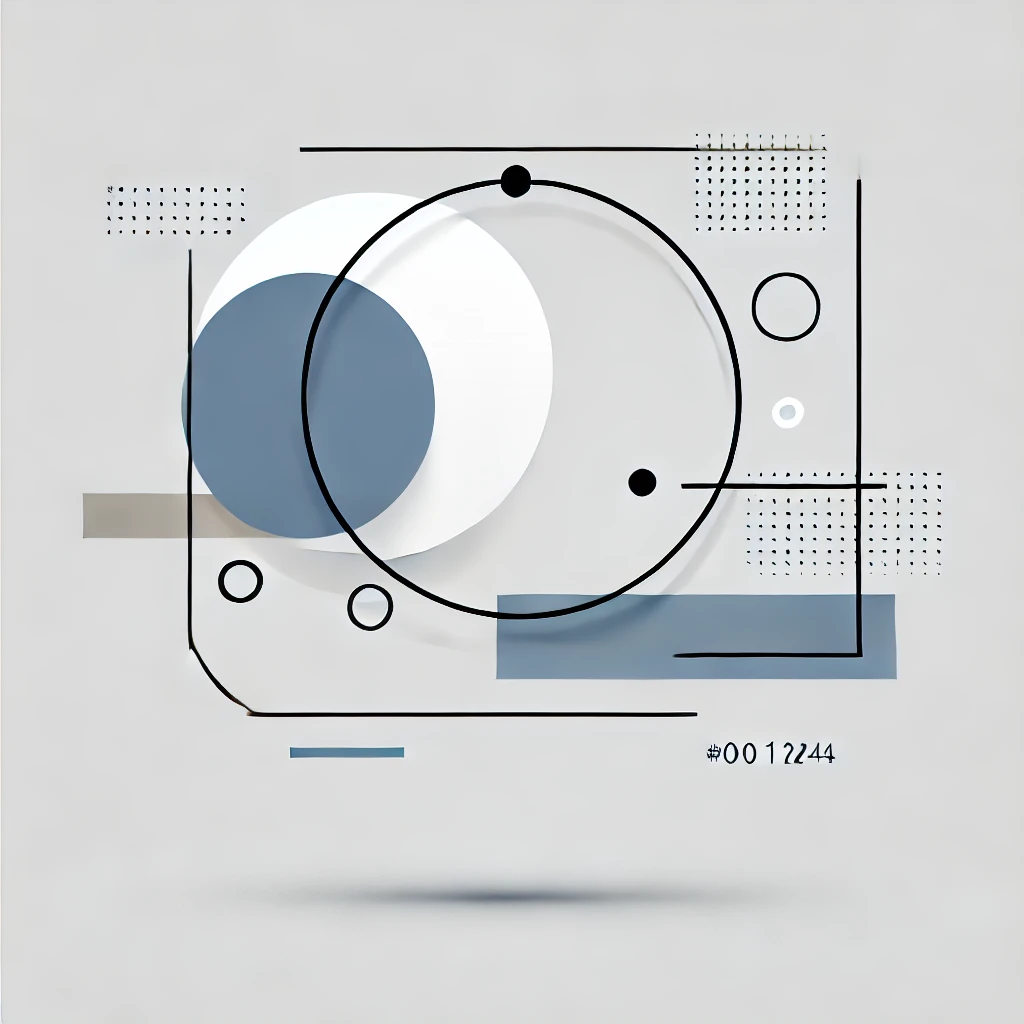
Newsletters
Analytics Advantage: The Essential Components of Data
Actionaut Shaun Davis edits “Analytics Advantage,” a weekly newsletter of actionable insights, proven strategies, and top tips for getting the most from your data and making high-stakes decisions with confidence. Here’s a sample issue. We hope you’ll subscribe.
The Essential Components of Data
Why It Matters:
- Timely data ensures your audience has what they need when they need it.
- Consistent data ensures your data is applicable to business questions.
- Accurate data builds trust in your product.
- Flexible data ensures your data stays relevant through time.
When I work with clients and discover that any of these principles are missing, my primary focus is to get them addressed and resolved. Only then can we start applying the data to the many questions they have and turning that data into actionable insights.
Intentional
Data needs to be intentionally designed. Start with the end in mind – consider the process, audience, and decisions you want to influence. Your data project will evolve over time, but at its core, every piece of data should have a purpose and address a business process or question.
There’s an important distinction between data capture and utilization. With cheap storage, capturing a lot of data for an unknown future use case makes perfect sense. Taking those raw materials and converting them to actionable data requires thoughtful planning.
The real value of data comes from when you can turn it into business insights, beyond storing and managing it.
We’ve all run across the data sources with 250 columns in them. This signals to me the designer tried to cover every possible use case or a product is bloated and needs redesign. In either case, the design usually lacks intentionality. Developers and consumers get overwhelmed and effectiveness lags.
Timely vs. Accurate
Timeliness is about the data arriving with enough time to make a decision. But there’s a caveat: speed comes at the cost of accuracy.
Let’s define some terms:
Timeliness: Data needs to arrive with enough time for the audience to use it to make a decision.
Accuracy: Data is within the tolerance for accuracy set by product owners. All data is an approximation of reality, so we have to set a tolerance for accuracy.
For your data to be timely, it needs to arrive on a consistent and predictable schedule so that people can build processes around it.
Example – Vehicle Crash Data
The example I always come back to is from one of my first data jobs: analyzing vehicle crash data. There are several different audiences that want to use information about vehicle crashes to make informed choices:
Law Enforcement: Law enforcement officers need timely data (yesterday, last week, or this month) to make daily operational decisions, such as resource allocation and officer deployment. The cost of a poor decision is limited to the time an officer spends running radar to address speeding.
Engineers: Engineers prioritize accuracy over timeliness; they are willing to wait months for data to be as accurate as possible. Their decisions, like infrastructure changes, are long-term and costly to undo (e.g., installing a roundabout). The cost of a wrong decision starts in the tens to hundreds of thousands or millions of dollars.
Finding Balance
When designing a dataset, consider:
The cost of a wrong decision: What’s the cost of a single employee’s time vs. a one-way decision which is hard to undo.
Audience needs: Different personas may require different levels of timeliness and accuracy.
Using the same dataset to serve different personas is possible and sometimes necessary.
Consistent
Creating consistent data is essential for building trust and ensuring accurate analysis. Consistent data is different from accurate data. It means internally consistent and consistent values in dimensions.
Internal Consistency
- Internally consistent data means that even if a particular measurement is off, it is consistently off.
This sounds odd, but going back to the principle that data is an approximation of reality, it makes sense. Data can never be 100% accurate. Knowing the quirks in data and ensuring those quirks are consistent leads to user trust. - Data should provide the same answer if the same question is asked twice.
If it doesn’t, it indicates a problem or that the data is changing over time, which can be confusing. For example, the number of people responding to a business offer will change as more people see and act on the offer.
Consistent Dimensions
Consistency in dimension comes down to quality, which is essential for how data is represented. For instance, a business name or street name can be written in various ways. It is important to standardize these representations within your dataset.
- Example: Martin Luther King Jr. Drive can be represented as “Martin Luther King, Jr. Drive,” “MLK Drive,” “King Drive,” or “MLK.” Creating processes to ensure these fields remain consistent is essential.
And so, when you’re designing your dataset, there needs to be a consistent set of ways that data is encoded and represented.
Flexible
Your business will change, and as a result, the questions you ask of your data are also going to change. Additionally, your understanding of a business process is going to change, which will change the questions you ask of your data.
Designing your data model with flexibility in mind is crucial. This means having some parts of your dataset that are purpose-built and others that are open-ended. Balancing these aspects ensures your dataset remains adaptable to changing business needs.
The Five Factors in Action
When working with clients, I find that datasets built with these parameters and principles are significantly more effective. Specifically, clients with such datasets:
- Gain a competitive edge and greater ability to apply data effectively.
- Have a much stronger understanding of their data.
- Possess a greater aptitude in applying data to a variety of questions and problems.
Addressing issues of timeliness, accuracy, consistency, and flexibility is essential before moving forward with data application, as each aspect directly impacts the trustworthiness and utility of the dataset.
When one or more of these parameters are lacking, addressing them becomes my primary focus.
Want to take action?
Book a Chat
Subscribe to Analytics Advantage
What are you waiting for?
The clock is ticking…
.